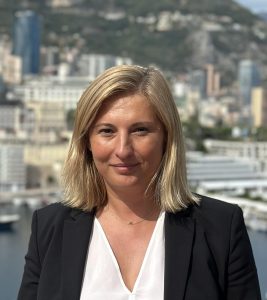
Interview conducted by Pascale Caron
Active Asset Allocation (AAA) is a financial engineering company specializing in designing bespoke asset allocation strategies, with a particular focus on risk management and actively limiting capital losses. The company has developed a patented methodology based on Maximum Drawdown, which is automated using artificial intelligence algorithms.
First, tell us about your business and what drove you to integrate artificial intelligence into your company?
I founded the company in 2010. At the time, we were developing investment algorithms for institutions, with an approach different from what was commonly done. Our risk management was based on maximum capital loss, and our models were dynamic, designed for a forward-looking environment. This allowed us to create strategies geared toward the future rather than simply analyzing the past.
Another key aspect of our approach was placing the client’s objective at the center of the algorithm, which was rare almost 15 years ago. Back then, few people asked what objective an algorithm or financial product should achieve. We persisted with this approach, which led to great success. Today, we advise over €22 billion in assets for major institutions, such as pension funds and mutual insurers. We work both on their own accounts and in creating distributed products, notably through life insurance contracts. This truly represents the DNA of Active Asset Allocation.
We then consolidated our financial engineering expertise into a digital platform aimed at serving savings actors within a digital ecosystem. This is how we launched our tool called PMS. It targets asset management companies practicing guided or delegated management, as well as insurers who integrate it into their life insurance contracts. It allows for portfolio construction, simulations, investment optimization, and report generation. It meets the growing needs for digital and efficient management in the savings sector.
A third aspect of our business is dedicated to savings distribution. To this end, we developed simulators for distribution actors, integrating sales support tools for tailored, often complex solutions. These enable clients to visualize the impact of monthly savings over several years when investing in a specific product or strategy, thus facilitating their decision-making.
Current regulations, particularly the Green Industry Law, push us in this direction. We are required to meet strict standards, and this is where our agility as a Fintech gives us an advantage.
Our systems are based on recent technologies like cloud-native solutions, and all our calculations are done via APIs. This approach is much simpler than for companies that have to manage technological layers accumulated over decades.
How did you approach integrating AI?
Hala: When I joined AAA six years ago, we were in full transition. At the time, we primarily conducted studies for our clients. We encapsulated our expertise into digital methods to meet our own needs and those of our clients.
We then sought to address various use cases by structuring and standardizing our approaches to make deployment simpler. We used the latest technologies, paying particular attention to data security, which is crucial when handling sensitive information. Over the years, these reflections allowed us to develop our digital platform.
We launched the first version in 2018, which was developed externally at the time. During the COVID period, we decided to revamp it, notably in July and August, and delivered it to our first major clients. For example, 1,200 MAIF advisors used our platform.
Today, we have a team of around 20 people. What is remarkable is that most have been with us for six years, and over time, we have built a team with unique expertise. We have also recently incorporated new recruits.
As for integrating AI, it has been part of our DNA as a Fintech. What has changed is the interconnectivity and advances that make AI more accessible and relevant. From the beginning, we used simulation and learning models, long before AI became a central topic of discussion.
Our algorithms do not rely on big data or generative AI but on a branch focused on specific calculations. This allows us, as solution providers, to deliver more speed and accuracy in our calculations, as well as more convergence and individualization. We also eliminate errors and automate repetitive tasks. Back then, it took us a week to work on two or three clients. With the growing demand from many clients, AI helps us make these processes much more efficient.
Do you use LLMs?
Currently, we do not directly use large language models (LLMs) in our core business, but it is something we are exploring.
However, we do occasionally use tools like Notebook LM, mainly to assist in writing content for marketing and communication, among other things. We have implemented strict rules for their use. For example, we favor paid versions with subscriptions managed by our CTO, and we remain very vigilant about data management. Additionally, we ensure that no sensitive documents are included and only manipulate public data that is intended to be seen by everyone, such as those contributing to AAA’s visibility.
We have seen the immense potential of this technology and the successes it has already enabled. It would be a shame not to take advantage of it! That’s why we have started exploring more advanced solutions, with ongoing projects to include LLMs in our business.
For example, we are currently conducting workshops to integrate small LLM-based models. These models will be trained on a relevant dataset to meet our specific reporting needs. Their performance will be optimized through fine-tuning and continuous adjustments, which will be based on scoring and the confidence level of the results provided by the models.
Have you noticed positive changes in your clients since you integrated AI?
Our clients often ask for detailed justifications of our models and their behavior at various stages. We strive to make this information accessible and understandable, to explain their workings precisely. Everything is transparent—there is no black box—, which enhances clients’ trust. Some were initially reluctant, but once they understood the system, they became much more open to AI.
Historically, we were more focused on institutional clients, but AI has allowed us to get closer to the quasi-mass market, although we do not work directly with end clients. For instance, we provide tools to financial advisors so they can offer tailored investment solutions. Our software simultaneously takes into account numerous parameters, such as the investment universe, management rules, risk levels, ESG criteria, and taxonomies, to generate a portfolio in seconds. This portfolio is then compared to the client’s objective to provide a suitable response.
This allows advisors to offer individualized solutions, even to two clients with similar economic situations. Each will receive a different portfolio, perfectly adjusted to their specific goals.
What advice would you give to SMEs or other companies hesitating to adopt AI?
It is crucial to be interested in AI, as it will be unavoidable in the future. Get familiar with the technology beforehand and clearly identify the use case you want to solve. Understanding the issues and risks is critical before diving in. Calling on experts can be wise, especially to avoid pitfalls related to infrastructure costs, the cloud, and data security. A poor technological choice can quickly become a budgetary headache. Adopt a pragmatic approach: evaluate the expected benefits against the costs and progress step by step, without expecting immediate revenue results.
Subscribe To Receive The Latest News
Curabitur ac leo nunc. Vestibulum et mauris vel ante finibus maximus.
Add notice about your Privacy Policy here.
Related Posts